當專案已經準備要導入Cassandra時,可能是專案已經遇到某些困難與瓶頸,並已經考慮採用Cassandra是一個正確的使用情境。以下是使用Cassandra: The Definitive Guide, Third Edition其中第十五章的部份段落來說明如何將資料表轉移到Cassandra上。
要將關聯式資料庫轉到Cassandra上,第一個遇到的問題就是如何將原本的資料表關係轉移到Cassandra上。一種方式是將既有的資料模型作直接轉譯(Direct Translation),以下會舉旅館預約系統來說明如何對資料模型作直接轉譯。
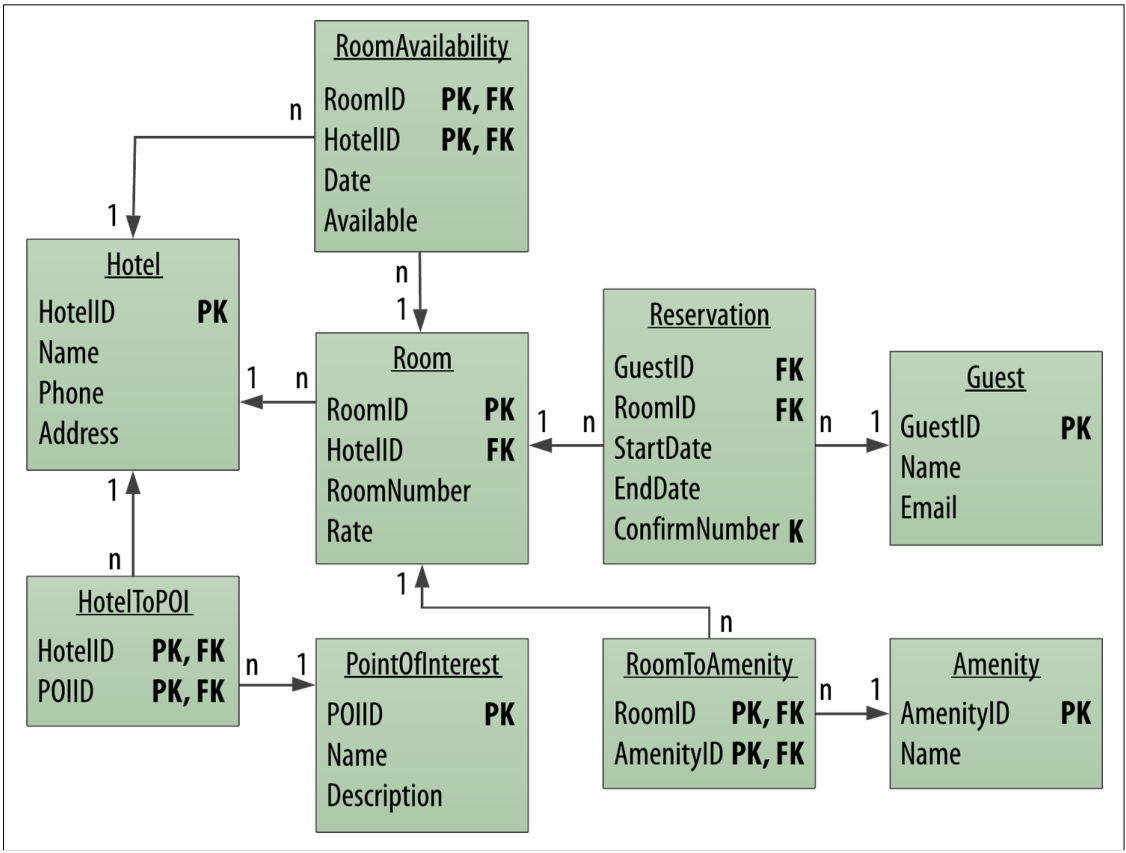
在將關聯式資料庫的資料表直接轉譯到Cassandra上,會分成二個部份:
- 轉譯實體(Translation Entities)
- 轉譯關聯(Translation Relationships)
轉譯實體(Translation Entities)
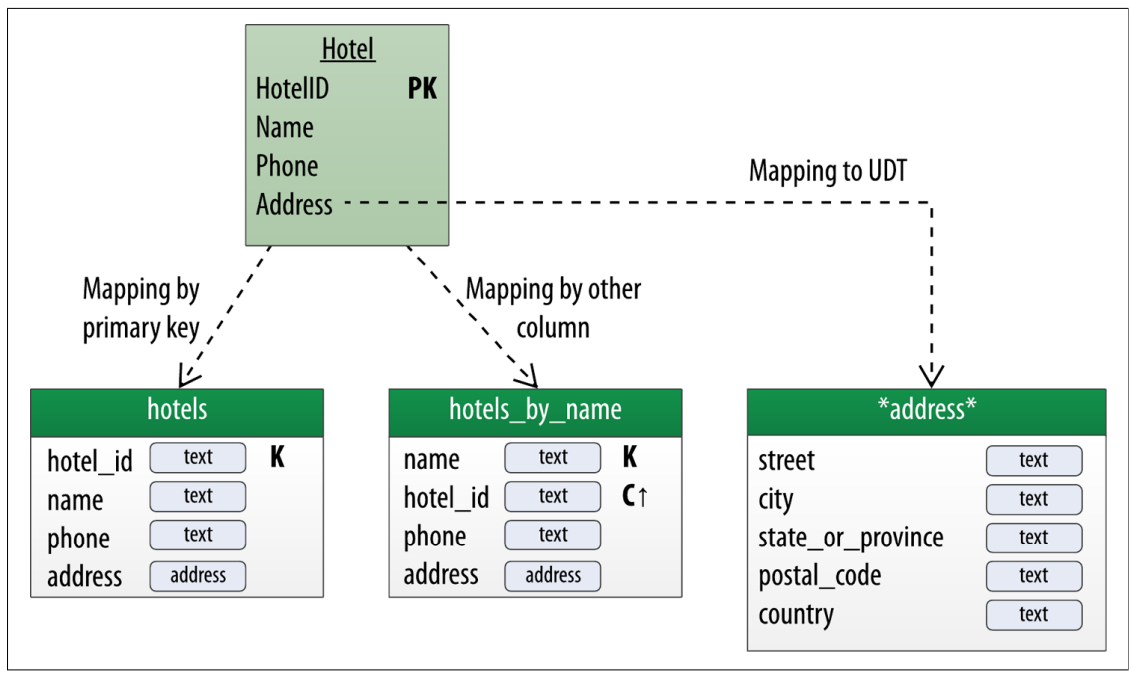
- 根據主鍵設定建立相似鍵值的Cassandra資料表
首先找到原本關聯式資料模型中的實體。比如說Hotel這張資料表會被預約系統查詢使用,這時就可以在Cassandra也建立一個具有相似鍵值的資料表,即為上圖的hotels資料表,其中參照原本的設定將hotel_id設定成主鍵(在這裡亦為partition key)。 - 建立反正規化資料表滿足不同查詢組合
因為並不是所有的查詢都是透過hotel_id查詢,假設要滿足使用name來查詢hotel資料的行為,那就需要建立另一張以name欄位為partition key的資料表叫hotels_by_name。這裡的主鍵為name和hotel_id組合,其中hotel_id為clustering key。 - 使用UDT(User-defined Type)描述複雜的型別資料
在資料表中的address可以是一個單純的字串表示地址,也可以是一個自定義的型別。因為在Cassandra中可以透過自定義型別來描述複雜的型別資料,以自定義型別Address來說,其中可以包含street、city等多個欄位的屬性。自定義型別並不是必須的,可以依系統的設計需求來考慮是否採用。
轉譯關聯(Translation Relationships)
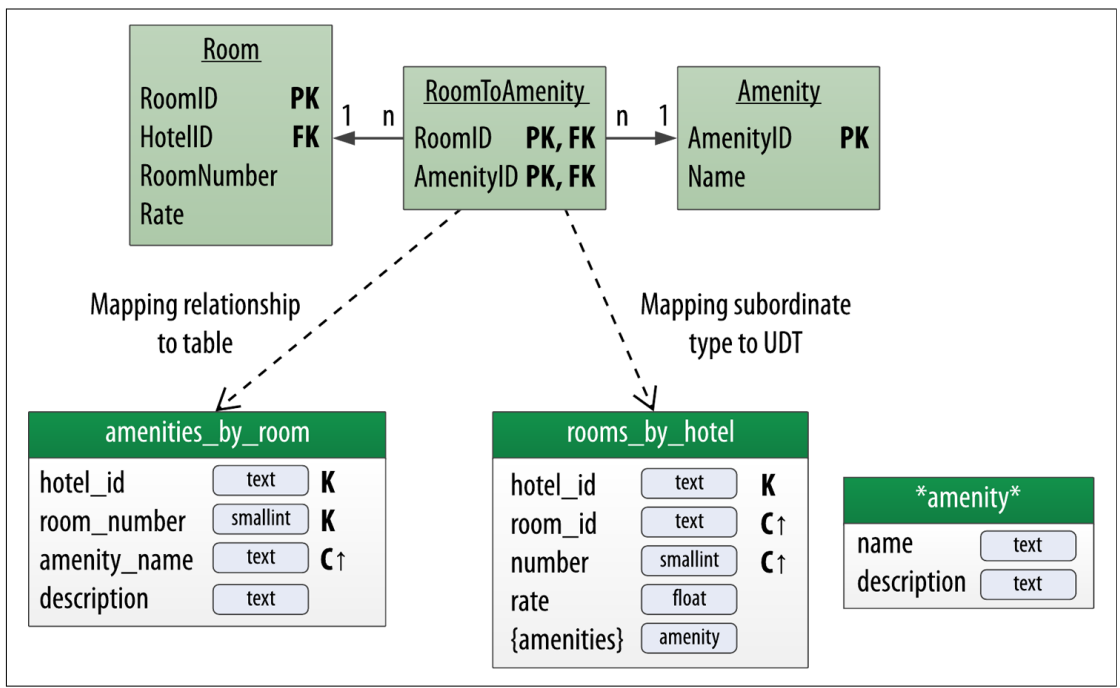
將實體的資料表轉譯到Cassandra的資料表後,接著就可以開始看這些實體資料表間的關聯性。以RoomToAmenity來說,這張為其實是描述Room和Amenity之間的關聯,而這類的關聯資料表常常沒有自己的屬性,可以看到RoomID和AmenityID這兩個欄位組成的主鍵,同時也分別是連接到Room和Amenity的外部鍵。
- 將實體資料表間的關聯性建立Cassandra資料表
這些被用來表達實體間關聯的資料表,時常伴隨著實體資料表作使用。以amenities_by_room為例,這張資料表的主鍵會拿實體資料表中的鍵值作為查詢用的partition key(hotel_id, room_number)。因此在查詢時就可以從hotel_id查詢某一間room_number提供的amenities。 - 使用UDT描述從屬資料
以右下角rooms_by_hotel資料表為例,這張表是透過hotel_id查詢所有的room資料,這時可以設計一個amenity自定義型態,並設定amenities欄位是list of amenity型態。這種設計的好處在於可以透過一個查詢就一併查出room相關的amenities資料,不需要分多次查詢。當然UDT的使用也是依系統的設計需求來考慮是否採用。
以上就是採用直接轉譯的方式將原本關聯式資料表轉成Cassandra資料表,這種方式好處在於可以直接對準在原本的資料模型作轉移,一步一步從實體資料表、資料表關聯還有結合應用層的查詢條件將專案作轉移。